Toothbrushing monitoring
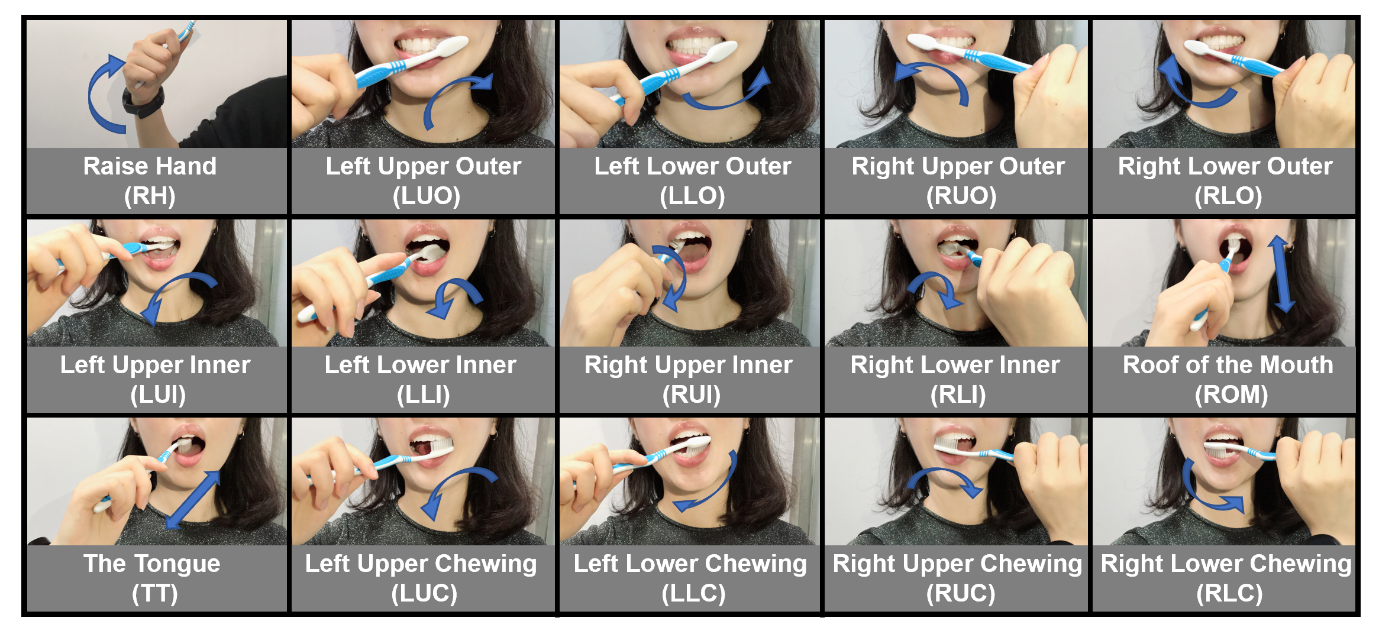
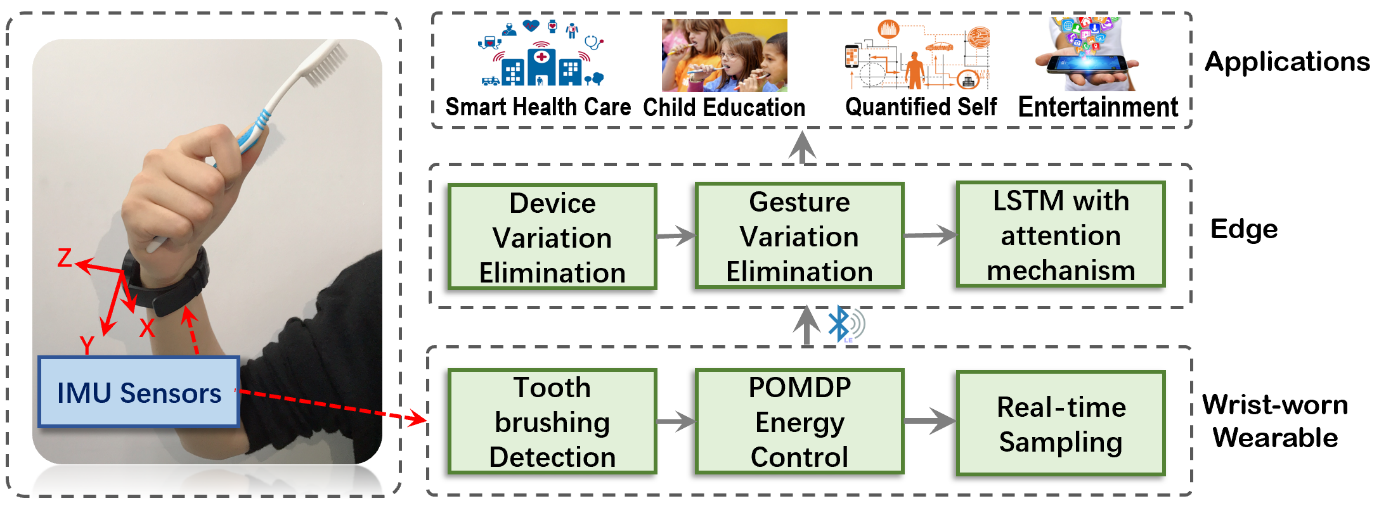
Oral health has significant impact on people's overall well-being. While many activity recognition systems exist in the literature, accurately sensing toothbrushing activities remains an unsolved challenging problem due to the diversity of toothbrushing habits among different users and subtle distinctions between different brushing actions. In this work, we propose Hygiea, an energy-efficient and highly-accurate toothbrushing monitoring system which exploits IMU-based wrist-worn gesture sensing using unmodified toothbrushes. To address toothbrushing variety, Hygiea incorporates a number of novel signal preprocessing techniques to automatically transform the sensory input during arbitrary toothbrushing activities to the consistent user coordinate system. To distinguish different brushing actions, Hygiea leverages an emerging deep learning model (e.g., AT-LSTM) to achieve fine-grained activity recognitions. Moreover, a POMDP model is incorporated for sampling control to balance activity detection and energy efficiency. Extensive real-world experiments show that the Hygiea system achieves a 11.7% accuracy gain compared to the state-of-the-art while maintaining energy-efficiency and zero modification on the toothbrushes.